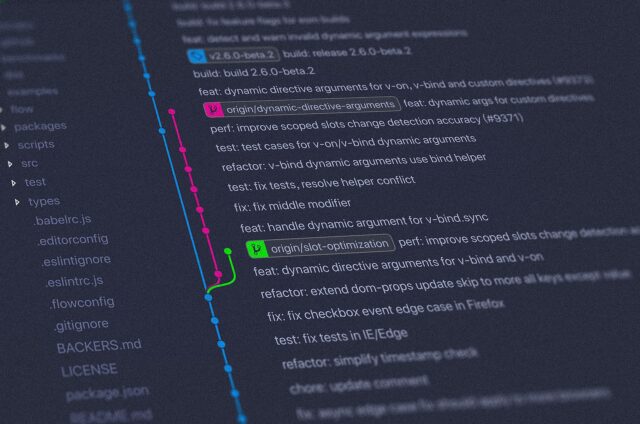
We are all used to relying on machine learning in everything: from surfing the internet to healthcare. ML solutions make accurate predictions, help to optimize work processes and reduce the workload. However, is this technology that harmless? Let’s find out.
1. False correlations
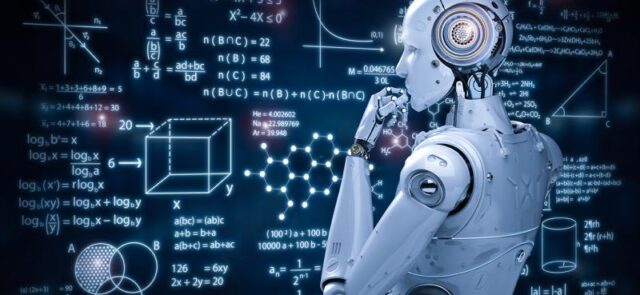
It is often said that machine learning is looking for patterns or correlations in data. However, usually, for example, in the case of regression analysis, false correlations might occur. A famous example is when Hathway stocks started to go up because many people were googling Hathway. They were googling the famous actress Ann Hathway after her new movie went out, but the machine didn’t understand it. If you want to learn more about correlations in ML, continue reading on the Serokell blog.
2. Machine learning affects people.
Remember any machine learning system that helped you to choose a movie. For example, Netflix offers you new movies to watch based on what movies you’ve already watched, how you rated them, and by comparing your tastes with those of other users. This way, the system can recommend a movie that you will most certainly enjoy.
Simultaneously, relying on artificial intelligence will change your tastes over time and make them narrower. Without the system, you would watch both bad films and choose films of unusual genres from time to time. But the course always recommends the safest bet. As a result, you cease to be a film expert and become only a consumer of what is given to you.
3. Ethics are challenging to formalize
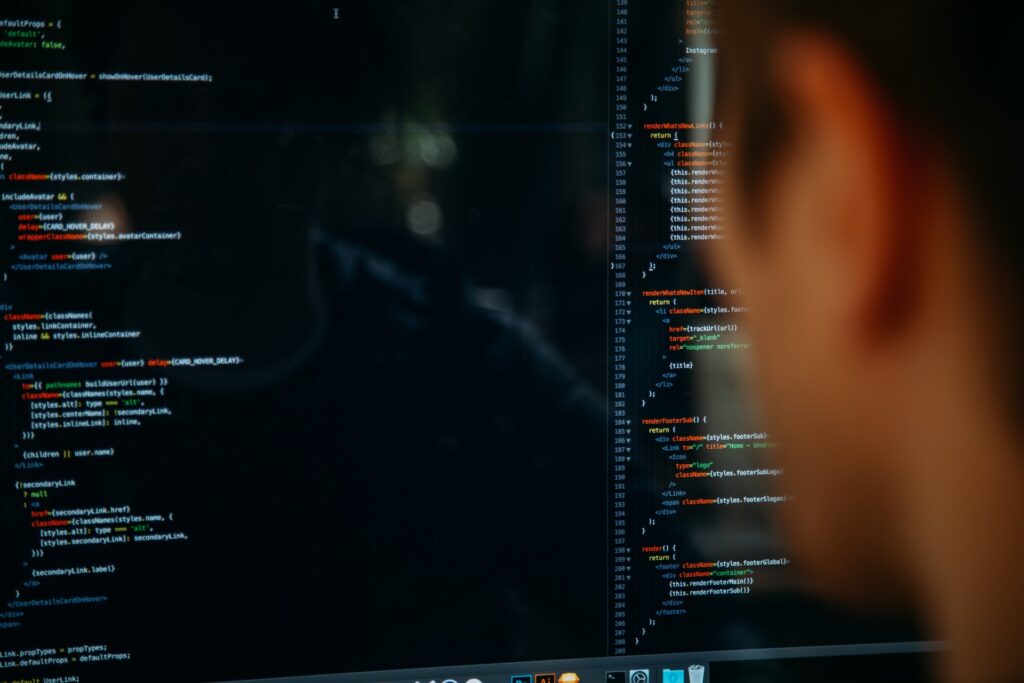
There is one problem with ethics that it is difficult to formalize. First, ethics change rather quickly over time. For example, society’s opinion on such issues as LGBT rights or feminism can change significantly over the decades.
Second, ethics is by no means universal: it differs even in different groups of the population of the same country, not to mention different countries. For example, in China, monitoring citizens’ movement using surveillance cameras and face recognition is considered the norm. In other countries, the attitude towards this issue may be different and depend on the situation.
Another pool of ethical problems is connected to the question of responsibility. Right now, Google, Tesla, and other companies are working on creating fully autonomous cars. So far, there have been no accidents involving such vehicles, but who to blame if a machine would kill someone? Dangerous situations can occur in different settings; for example, what if there will be a bug in a smart home system or chirurgical software?
4. Wrong causes
Usually, the creators of machine learning algorithms don’t want to cause any harm, but they want to earn money. This can cause some problems: for example, now we can see that ML models created to process texts and help professionals are used to create fake news. It is a big question whether the creation of such programs was a good or an evil deed because, generally, humans are quite bad at detecting fakes created by such machines. In the meanwhile, they can affect people’s lives a lot, manipulating stock prices or politics.
5. Lack of data
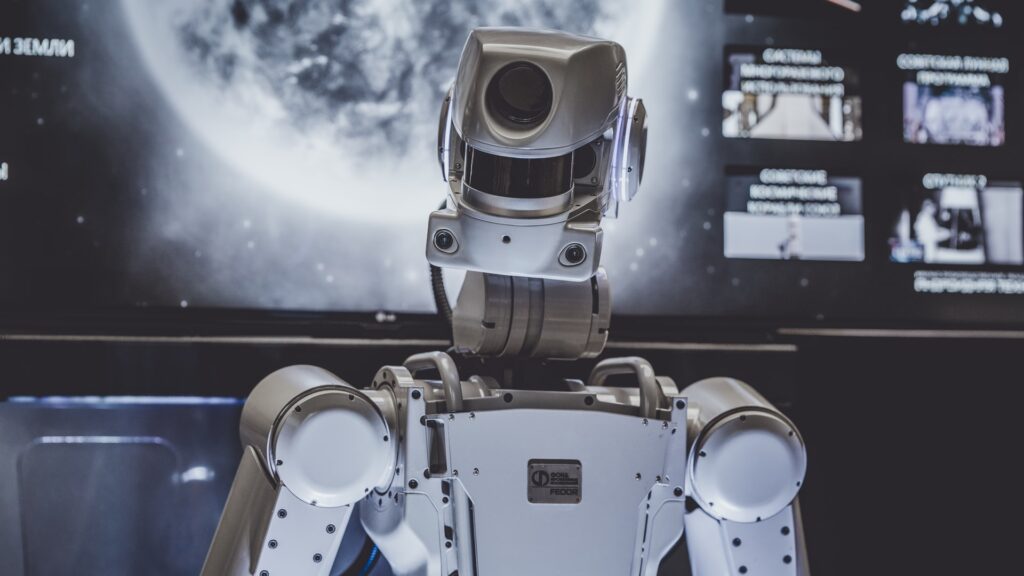
It is one of the trickiest tasks in machine learning to find and collect reliable data. This process is expensive and time-consuming, so programmers often have to operate in situations when there is not enough data.
Simultaneously, many machine learning algorithms need a lot of data to learn from if you want them to be accurate. This is especially true for DL algorithms, such as neural networks. They become better at their predictions the more data they get during training.
6. Noisy data
Training the algorithm strongly depends on the initial data based on which the training is conducted. The data can turn out to be wrong. This can happen either by accident or by malicious intent (in the latter case, this is usually called “poisoning”).
Microsoft once taught a chatbot to communicate on Twitter, based on what other users were tweeting. The experiment had to be closed in less than a day because the internet users quickly taught the bot to swear, hate women, gays, and Jews, and quote “Mein Kampf.”
7. Interpretation problem
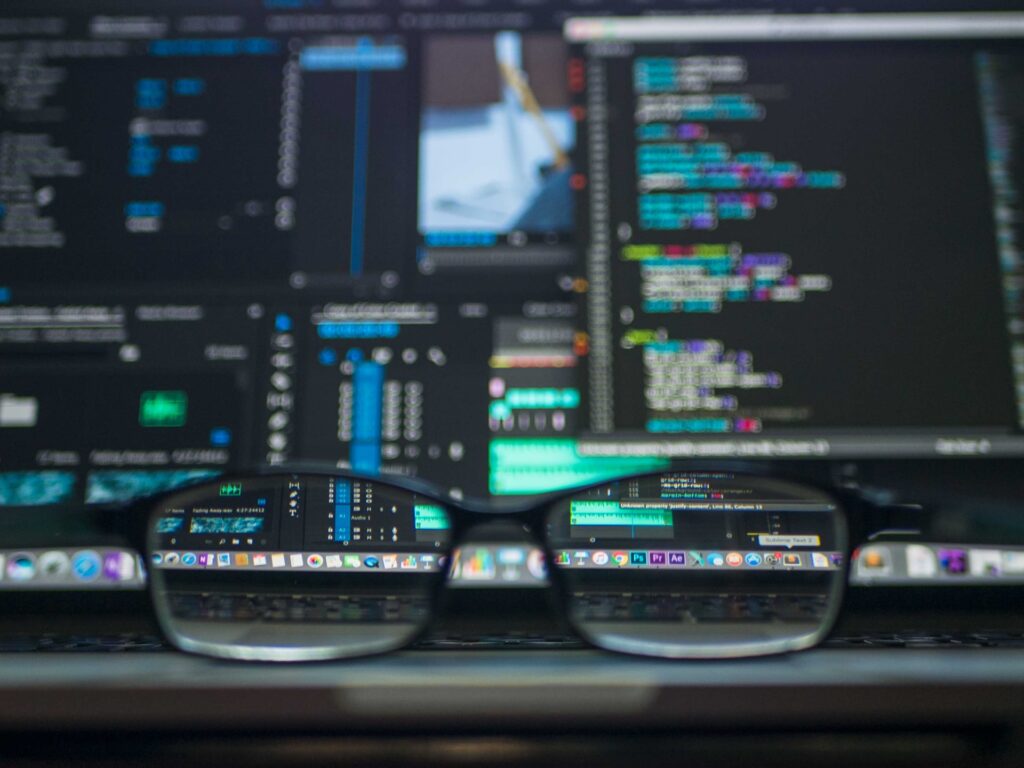
When working with machine learning, especially deep learning models, the results are hard to interpret. For example, one can apply AI to solve their client’s problems and get some results. But a DL algorithm is a black box. How can they prove to the client that their products are accurate if they do not know the logic behind this decision?
This limitation of machine learning sometimes repulses business people. They prefer to address a traditional human consultant who can provide reasons for their conclusions. Hopefully, this problem will be solved in the future, and people will learn to interpret neural networks.
8. Hacking and poisoning
Poisoning is impacting the machine learning process. But it is also possible to deceive a ready-made, properly working mathematical model if you know how it works. For example, a group of researchers managed to learn how to deceive the face recognition algorithm using special glasses that make minimal changes to the picture and radically change the result.
This is a harmless event, but it means that he can trick them while a human is more intelligent than the machines. Using this technique, one can prevent scanners from finding potentially harmful items in their airport bag, for example.
Similarly, a hacker can interfere with the system and produce wrong results by changing the input data. We will not fully trust ML until we figure out how to deal with these problems.
Conclusion
We will rely more and more on machine learning in the future only because it will generally do a lot better than humans. Therefore, it is essential to remember the shortcomings and possible problems, try to foresee everything at the stage of systems development – and do not forget to keep an eye on the algorithms’ results in case something still goes wrong.